Table of contents:
- Introduction
- Core Functions of Business Intelligence
- Common BI Tools and Technologies
- What is Data Analytics?
- Different Types of Data Analytics
- BBusiness Intelligence vs. Data Analytics
- Common Mistakes Businesses Make with BI and Analytics
- Conclusion
Introduction
Businesses in the present data-rich environment deal with massive information volumes. Businesses use business intelligence (BI) and data analytics as main concepts to leverage their data pool effectively. The related concepts both possess distinct roles because they deliver unique perspectives from each other. Organizations that recognize the differences between these two frameworks can use this information to make better decisions that lead to business expansion.
Core Functions of Business Intelligence
Business Intelligence activities cover the assembly, unification, and delivery of business data, analytic work and output delivery. The primary purpose of Business Intelligence is to build comprehensive organizational performance visibility to guide better business decisions. Through BI, organizations can detect trends while tracking KPIs before creating reports summarizing historical business information. BI systems convert raw data into significant insights, allowing stakeholders to base their choices on data.
Common BI Tools and Technologies
Several tools and technologies are commonly used in Business Intelligence, including:
- Tableau: A powerful visualization tool that helps users create interactive dashboards.
- Power BI: Microsoft’s analytics service that provides business insights through visualizations.
- QlikView: A data visualization and dashboarding platform that allows for associative data exploration.
- SAP BusinessObjects: A front-end application suite allowing business users to view, sort, and analyze business intelligence data.
These tools facilitate the process of gathering and visualizing data, making it easier for organizations to derive actionable insights.
What is Data Analytics?
Data Analytics goes a step further than Business Intelligence. It analyzes historical data and applies statistical techniques and algorithms to uncover patterns and predict future trends. It also analyzes data symbols and their origins to understand the information better. By using data analytics, organizations better understand customer actions and optimal system operations, resulting in improved organizational outcomes.
Different Types of Data Analytics
Data Analytics can be categorized into several types:
- Descriptive Analytics: Focuses on summarizing past data to understand what has happened.
- Diagnostic Analytics: Investigates past performance to determine why something occurred.
- Predictive Analytics: Uses statistical models and machine learning techniques to forecast future outcomes based on historical data.
- Prescriptive Analytics: Suggests actions based on predictive analytics to optimize outcomes.
Each type serves a specific purpose and can be leveraged depending on the organization’s goals.
Business Intelligence vs. Data Analytics
While both Business Intelligence and Data Analytics aim to improve decision-making through data, they differ in their approach:
- Focus: BI primarily looks at historical data to provide insights about past performance, while Data Analytics seeks to predict future trends and behaviors.
- Techniques: BI relies on querying and reporting tools for analysis, whereas Data Analytics employs statistical methods and algorithms for deeper insights.
- Outcome: BI provides a snapshot of what has happened in the business, while Data Analytics offers recommendations on what could happen next.
Understanding these differences is crucial for organizations looking to leverage both effectively.
Common Mistakes Businesses Make with BI and Analytics
Many businesses fall into common pitfalls when implementing BI and analytics solutions:
- Lack of Clear Objectives: Without defined goals, measuring success or determining which metrics matter most is challenging.
- Ignoring Data Quality: Poor-quality data can lead to misleading insights; ensuring data accuracy is essential.
- Overcomplicating Tools: Overly complex tools can hinder user adoption; simplicity is key for practical usage.
- Neglecting User Training: Failing to train staff to use BI tools or interpret analytics can result in underutilization.
Organizations can avoid these mistakes by maximizing the value derived from their BI and analytics initiatives.
Conclusion
Modern data strategies require business intelligence and analytics, although these two components fulfill separate functions within the decision-making process. The main difference between these two analytics methodologies is that BI extracts historical insights to understand performance, whereas data analytics utilizes statistical methods for trend forecasting. Businesses can maximize the power of strategic decisions by combining these two approaches and understanding their specific functions to reach their objectives in a competitive market.
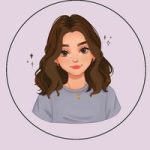